A sustainable deep learning framework for fault detection in 6G Industry 4.0 heterogeneous data environments
Peer reviewed, Journal article
Published version
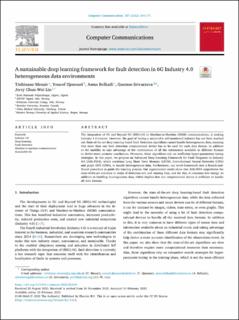
Åpne
Permanent lenke
https://hdl.handle.net/11250/3025660Utgivelsesdato
2022Metadata
Vis full innførselSamlinger
Originalversjon
Mezair, T., Djenouri, Y., Belhadi, A., Srivastava, G., & Lin, J. C.-W. (2022). A sustainable deep learning framework for fault detection in 6G Industry 4.0 heterogeneous data environments. Computer Communications, 187, 164-171. 10.1016/j.comcom.2022.02.010Sammendrag
The integration of 5G and Beyond 5G (B5G)/6G in Machine-to-Machine (M2M) communications, is making Industry 4.0 smarter. However, the goal of having a sustainable self-monitored industry has not been reached yet. State-of-the-art deep learning-based Fault Detection algorithms cannot handle heterogeneous data, meaning that more than one fault detection computational device has to be used for each data format, in addition to the inability to take advantage of the combination of all the information available in different formats to derive more accurate conclusions. Moreover, these algorithms rely on inefficient hyper-parameters tuning strategies. In this paper, we propose an Advanced Deep Learning framework for Fault Diagnosis in Industry 4.0 (ADL-FDI4), which combines Long Short Term Memory (LSTM), Convolutional Neural Networks (CNN) and graph CNN (GNN), to handle heterogeneous data. Furthermore, our novel framework uses a Branch-and-Bound procedure to guide the learning process. Our experimental results show that ADL-FDI4 outperforms the state-of-the-art solutions in terms of detection rate and running time, and for that, it consumes less energy. In addition to handling heterogeneous data, which implies that one computational device is sufficient to handle all data formats.